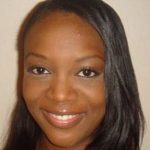
Sponsored Article – Author: Pierre Guyot, Editor, Usbek & Rica
The symposium “New Generation of Networking Innovation and Research” Cisco – École Polytechnique 2017
What is the current situation of Artificial Intelligence (AI)? “In an extremely interesting place in its development!” answers Ben Goertzel, a man of many companies and evangelist for the concept of general artificial intelligence. The question of the value of AI for businesses having been settled, we are today in a phase of technological transition.
The “general artificial intelligence” of Ben Goertzel is that which aims to go beyond the learning process of artificial neural networking (the famous “deep neural networks”), this sub-domain of AI that according to him “has become extremely popular for it solves very demonstrative problems: facial recognition, language processing, automatic translation, and even the game of go,” but turns out to be a form of “narrow artificial intelligence.” This narrow AI is in fact “only” capable of identifying patterns in large sets of data, but not of reproducing the much greater spectrum of human intelligence, which has to do with perception, reasoning, emotion, empathy, creativity, etc. Turn around the board game of go and narrow artificial intelligence can no longer beat man!
General artificial intelligence and the network of the future
What still makes Ben Goertzel optimistic is that after having exchanged with a great number of network researchers met during the symposium, it seems to him that they “share the same intuition as [he] about the right direction to follow for AI research in the future: neural networks are precious and will be part of the solutions, but other ideas and other architectures will need to be integrated.”
Up to the point of making network infrastructure an AI in itself? Ben Goertzel believes that, in any case, general artificial intelligence is in accordance with the philosophy that underlies Information-Centric Networking (ICN), that is, that of a vast distributed network of artificial intelligence and of cognitive multi-agent systems. Furthermore, ICN and AI complement each other: in broad outline, with ICN, information is no longer addressed only by its content, and so services and programs that function on the network can then be distributed from any point on it. The AI can then be used to determine the best point from which to deliver these services. While waiting, and taking place now, AI already allows the improvement of network security and the efficiency of information transport and its management by human administrators.
Nevertheless, the open source platform, developed by Ben Goertzel, manages very complex information graphs, having to do with cognitive algorithms, each acting on a different type of knowledge but in the same common space. These “graphs,” which find their use in medicine, robotics, and finance, and which can prefigure the future of AI, also needs a more complex network architecture: cognitive, integral and “neuro-symbolic,” not attached to a particular task but conceived for autonomous access and for unpredictable environments, thanks especially to symbolic reasoning methods.
“In the end, with information-centric networking, the whole network is going to become a kind of artificial intelligence” – Video interview with Ben Goertzel, president of Novamente LLC
The needs in infrastructure for machine and deep learning
It is to another researcher in artificial intelligence, Andrew Ng, that James Jones, client solutions architect for Cisco, refers to when the time comes to evoke the challenges in architecture for the rollout of machine learning and deep learning on a grand scale. In effect, according to this researcher from Stanford, he preached in 2008 that deep learning should use the capacities of graphic processors (GPU), which he has since achieved for the most part: “It is now the time for machine learning to adopt High-Performance Computing (HPC).”
But what are the current needs of AI in infrastructure? From about ten years ago, the big artificial intelligence industrialists, great demanders of power for calculation, have gone forth to research the means of accelerating even more the processing of their operations, and in particular the training of their neural networks. They rely mostly on GPU’s to do this, particularly AlphaGo, the Google program that had defeated man in the game of go in March 2016. This GPU wave, launched in 2006, is not ready to stop: the constructor Nvidia has 13 times more partners in this domain than two years ago, and is recruiting in the same period three times more GPU developers. But the industrialists also currently rely on intelligent chips (IPU), more naturally dedicated to this type of calculation, and, finally, since these processors alone are not enough, on external memory: virtual machines authorizing advanced computational capacities in the cloud, with a considerable number of calculation nodes, allowing, with a multiplicity of instances, to scale its tasks at a distance.
Rémi Coletta and Benoît Gourdon, respectively data scientist and CEO of the start-up of predictive connected objects, Tell Me Plus, also agree: the progress of AI is exceptional. And, to obtain a successful predictive AI system for the end-user (whether an individual using advanced domotics or a company using wind turbines and wanting to put in place predictive maintenance), one must use AI. This will optimize the architecture and the energy used for calculation. Machine learning is especially used here, including even embedded machine learning, accessible in the cloud from a connected terminal. This is a vast field of applications for tomorrow’s AI companies.
Martin Raison, engineer for Facebook AI Research, the Facebook entity dedicated to artificial intelligence, and which opened a headquarters in Paris in 2015, evokes a need for “virtually unlimited” resources: the hardware platforms progress in this domain, as much in terms of storage as of efficiency, but the needs continue to increase in parallel. As long as these remain ensconced in what Ben Goertzel calls “narrow” artificial intelligence, they are constrained by the environment and must limit themselves to a finite number of accessible informational data. But even in this field, the size of the models used to train the neural networks does not stop growing: for example, Facebook has been looking only recently to have its algorithms respond to complex questions, which, for instance, needs reasoning from textual analysis of articles in the online encyclopedia Wikipedia.
Also, the techniques for optimizing memory required for deep learning are diversifying: certain techniques rewrite output data onto input data; others find dependencies between the two through calculation during data processing, and optimize memory as a result by reusing space that up to then was occupied by useless data. Still others over-optimize calculation capacity, or that of memory, thanks to, for example, neural networks, said to be recurrent. “GPU accelerators” coupled with CPU’s, which are also entering the market. As for the architecture, the clusters of deep learning use a great diversity of servers (up to eight nodes per cluster) in order to respond to all the needs. Finally, the time has come for hyper-convergence: processing, storing, networking and virtualization all operating on the same platform, allowing deep learning to speed up whatever the framework being used. The new supercalculators dedicated to deep learning, such as Saturn V, clearly seem to correspond to the wishes of Andrew Ng, and a standard proposed by various manufacturers, defining a GPU server adapted to data center supercalculation, is even on the way to be defined.
Download the presentations of the speakers in this session
Relive the symposium now by looking at our playlist.
Find all the symposium articles on our blog: :
- The Future of Infrastructures: Exceeding the Limits
- The Opinion of Mark Townsley, Cisco Engineer and Co-founder of the Paris Innovation and Research Lab
- Information-Centric Networking: the Infrastructure of Tomorrow?
- Media Production Makes its Transformation to IP
- IPv6-centric Networking, a Crucial Rollout for Tomorrow