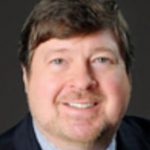
I’ll never forget a high school teacher telling me a truly new idea was the rarest thing on earth. I thought about that concept recently when I happened across the latest edition of Gartner’s Top 25 Supply Chains report (Cisco is #7 in the world; the only Data Centre vendor in the top 10). The report reminded me how dramatically the industry has changed during my career, and yet how many of our core manufacturing IT concepts have remained essentially unchanged.
In the very first Top 25 Supply Chains report in 2004 my company was ranked #1, largely based on our use of RFID tags in our manufacturing division. We didn’t know this was early IoT. We just thought RFID was a convenient way to track inventory from our manufacturing and distribution facilities, to our retailers’ shelves, via our 3PL partners; industry standards made the tags universally readable by all the participants in our route to market. Easy. RFID had the added benefit of generating consistent data about sales and inventory which could be manipulated by the Data Warehouses of that era.
Good News, Bad News
Like its predecessor, barcodes, RFID tags are still widely used by Manufacturers. However, one reason RFID remains so popular – its universal, highly structured nature – is also its limitation. Structured data accounts for only about 20% of available data. The rest, usually the main focus of IoT initiatives, is unstructured, rapidly increasing in volume, and all over the board: buried in documents and emails, surfacing in customers’ social media posts, and in manufacturing, often created by disparate sensors, pumps, and motors attached to legacy machinery. Further complicating matters, unstructured data is often generated far from the predictable confines of a warehouse or a store shelf, in locations inconvenient for human observation.
The good news is there is more data out there than we ever imagined.
The bad news is there is more data out there than we ever imagined.
How does one efficiently process all this data and turn it into valuable insights without consuming every available cycle of your data centre?
Dynamic Tiering
One technique used to segment massive amounts of data and make it more actionable in real time, is Multi-Temperature Data Management, also referred to as Dynamic Tiering. This concept recognizes not all data is equal or needs to be addressed with the same level of urgency. It is especially true in the IoT era, where almost everything can be collected. But if some of the information is not critical, why expend the resources to move it to your big data processor and bog down your network with added latency and your analytics with superfluous data points? In this technique, data is usually divided into three tiers: Hot, Warm, and Cold.
- Hot Data is data that needs to be accessed most frequently and usually in real time. Therefore hot data should be processed on your fastest compute and storage platform, such as a Cisco UCS-based FlexPod All-Flash solution (with NetApp) or a UCS-based FlashStack All-Flash solution (with Pure Storage). Hot data is typically your most recent and mission–critical information: Retail POS transactions during the holiday shopping season is a good example. Or manufacturing inventory for a current production run.
- Warm data is less frequently accessed data, and may be stored on more conventional storage platforms. Quarterly reports for example, or other older data that may be relevant, but probably not needed for real-time business insights. It may even be a back-up instance of your current production environment, stored at a “warm” recovery location.
- Cold data is your least accessed data, sometimes even information that could be considered “noise” in the system. This can be stored offline, or in some instances even deleted to save money. Often however, cold data refers to information you must retain for long periods of time for business or regulatory compliance purposes.
Computing at the “Edge”
Edge Computing is another technique manufacturers can employ to maximize the efficiency of data processing in an IoT environment. Edge computing refers to an architecture that locates compute power right at the “edge of the network” in order to generate business insights faster, generally in close physical proximity to where the data is both collected and used. This may be a local appliance, a device such as programmable automation controller, or as in the example below, a smart camera with added intelligence built into it. This speeds responsiveness and reduces costs by avoiding the need to ship data back to a central processing location.
The term edge computing is sometimes used interchangeably with Fog Computing. Not to be too picky, but they are subtly different. Fog computing, pioneered at Cisco by the way, locates intelligence at the local area network level and processes the data in an IoT gateway or a “fog node.” Advocates of the fog approach argue it is more scalable and offers greater visibility across your network. While proponents of edge computing argue the architecture is simpler, with less layers, and therefore fewer points of potential failure. But both options offer the benefits of distributing traditionally centralized data centre intelligence, out to where the action is.
The Smart Train
Even I have to admit, after a while this all starts to sounds like vendor gobbledygook. So let’s review a real-world example from one of our customers, a European National Railway. We worked with the Railway on an IoT solution that moves computing to the edge of the network to improve their riders’ experience, increase safety, and simultaneously reduce costs.
First, the Railway deployed intelligent Cisco Meraki cameras in passenger cars to identify empty seats and transmit that information to terminals at the next station, allowing waiting passengers to reserve seats on platform kiosks. The intelligence to distinguish an empty seat from an occupied seat, is processed in the device itself – there’s no need (or time) to send that information back to a central data centre. The information on premium open seats is hot data and allows those seats to be sold on the spot for a fee. But once that seat is occupied, the information that the seat was open is of no value, and therefore that cold data is trashed. This is a great example of data migrating from Hot to Cold in literally seconds.
For added safety, the Railway deploys similar cameras underneath the passenger cars to inspect the wooden railway ties for damage or rot. Although these trains travel at up to 150 mph, the Cisco Meraki cameras look at every wooden tie and assess it for damage in real time. The locations of damaged ties are tagged with GPS and sent to the Railway’s road crews for repair. In the past it might take a year to inspect every railroad tie in the system, let alone replace them. Now however they are inspected every time a train passes. Not only does this create a safer transportation system, the Railway told Cisco they’re saving $1M every year on just the fuel for their old railroad inspection trucks.
Click here if you want to learn more about how Cisco Big Data and Analytics solutions can save you money and increase the efficiency and speed of your manufacturing data centre.
1 Comments
I'm interested to your article about the part of smart train.
I want to know more about whether this technology (MEC) is used in SJ Labs' "Hitta obokad plats" (refer to https://www.sj.se/sv/om/om-sj/sj-labs.html).
Thank you!