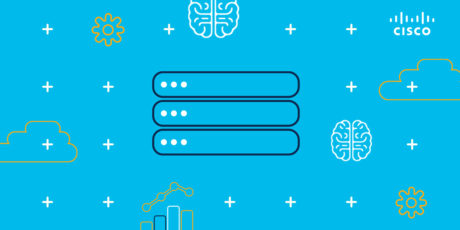
Rise of the machine learning and AI opportunity
What is the difference between a chihuahua and a muffin? There are many answers you could give, but would your IT infrastructure be able to distinguish between the two?
More importantly, is your IT infrastructure able to quickly flag an application performance issue, identify the root cause, provide you with recommended actions for remediation and implement them in automated fashion, while showing the impact of said issue and fault resolution on your business’s bottom line?
If artificial intelligence (AI), machine learning (ML) and the ability to demonstrate the value of IT innovation back to your stakeholders in real time are top of mind for you, watch this short video to see Cisco’s Chief Architect Carlos Pereira, and Cisco AppDynamics’ Director of Business iQ Matt Cleve do exactly that.
If the integration of AI, ML, and IT infrastructure to enhance your systems management and operations capabilities whets your appetite more than a muffin, then read on…
(No chihuahuas were harmed in the making of this blog!)
AI and ML 101
It’s easy for us to identify objects, animals, faces etc. because we learn from experience. Computers however, learn from instructions. We give them a program that tells them exactly what to do.
This is where AI and ML technologies come in. They allow computers, internet of things (IoT) enabled devices like cameras, and entire IT systems to learn from data.
AI is one of the top priorities for enterprise IT in every sector as businesses explore ways to extract more intelligence from the masses of data they generate each day. This intelligence can be applied to augment human capabilities and make better decisions, increase efficiency through automation and enhanced operations, and gain competitive advantage.
AI is an umbrella term applied to any technology that automates human-like tasks. In the majority of today’s AI projects, it’s ML that lies at their heart.
ML is based on the creation of a mathematical model, which is then fed training data in order to allow machines to learn from data and observations. In the case of our opening question, the training data could be hundreds, thousands, or even millions of pictures of muffins and chihuahuas.
Once our ML model is established and enables a machine to correctly distinguish between a muffin and a chihuahua, it’s fine-tuned automatically until it reaches a high level of accuracy. At this point, new data can be fed into the ML model in order to add new features and capabilities.
Impact of AI on IT infrastructure
Data scientists are the architects of ML-powered software and are fast becoming the rock stars of IT as the adoption of AI gains pace. Global AI revenues (including software, hardware, and services) are expected to hit $156.5 billion this year, according to IDC, and grow by 17% annually to surpass $300 billion by 2024.
Many businesses have already started with AI but are now looking to adopt it at massive scale, which means understanding how it will impact their IT infrastructure. AI is GPU-intensive and calls for powerful compute capabilities. ML algorithms can run on traditional x86 CPUs, but GPUs accelerate these algorithms by up to a factor of 100.
AI also calls for vast amounts of storage to house the data that fuels it (i.e. data lakes and Big Data environments). It will likely include deployments at the network edge, such as cameras or other IoT devices, and edge computing to enable faster processing of data locally.
Although data scientists typically prefer to use public clouds offering specialised stacks of ML software and tooling, deploying ML stacks on-premises assures data sovereignty and can make data access and processing more financially predictable. This is why AI is increasingly being adopted in hybrid cloud configurations that allow for prototyping in public cloud ML environments and data training on premises, with connectivity to network it all together.
AI Ops
There are many AI use cases today that fall under the auspices of competitive advantage. But the opportunity AI affords businesses to enhance their IT systems management and operations is just as compelling.
AI Ops refers to the way information from key data domains – i.e. Business, User Experience (UX), Application, Network, Infrastructure, Security – are managed by IT teams. In today’s business climate, every second of network infrastructure degradation or outage can adversely impact application performance and the user experience.
As the primary interface between a business and the consumer in the digital economy, the application is the non-negotiable KPI. If an application takes too long to respond, consumers will opt for an alternative. Research shows consumers are fast becoming more loyal to an experience than a brand.
AI Ops enables businesses to move from reactively resolving faults to proactively improving performance and user experience. This is a new mindset that involves transforming current IT operating models to better manage an increasingly distributed mix of legacy and new applications across an ever more diverse and extended enterprise environment.
There are 3 key pillars to achieving this AI Ops transformation:
- Visibility – e.g. business and application performance issue detected
- Insights – e.g. application performance issue correlated with underlying infrastructure constraints
- Action – e.g. constraint removed by right-sizing infrastructure environment
Show value of business outcomes
It’s essential that your IT teams can ensure applications run smoothly and deliver the optimum user experience. In addition to performing their day jobs, IT teams also need to strike the right balance between application performance and controlling cost both on-premises and in public clouds.
AI Ops is all about letting machines manage machines. Insights and automation powered by AI can provide your IT teams with the ability to optimise workloads effectively at “cloud scale and speed” and assure the best possible application experience.
Combine AI Ops with a management platform that gives you monitoring, policy management, hardware and software deployments, and IT operations automation in a single SaaS solution, and you have the ability to understand application performance and UX. If there is an infrastructural constraint (surfaced by insights), your IT team can remove that constraint quickly.
Crucially, AI Ops allows your IT teams to relate an issue back to the business and show key stakeholders the impact that the issue has had, empirically and in real-time, on your business’s bottom line.
You can learn more about AI, ML, and the impact of these technologies on your IT infrastructure with one of our resident gurus Roger Dickinson in his latest webinar: AI for Network Engineers (listed under Developer Advocacy).
Tags: